
Piano rolls are great for piano performances, because they are able to exactly capture the timing, pitch and velocity (i.e. Right: modern incarnation of a piano roll. Left: player piano with a physical piano roll inside. Much of the work on music generation using machine learning has made use of (some variant of) this representation, because it allows for capturing performance-specific aspects of the music without having to model the sound. This representation survives in digital form today and is commonly used in music production.

Holes were punched into a roll of paper to indicate which notes should be played at which time. This dramatically reduces the amount of information that the models are required to produce, which makes the modelling problem more tractable and allows for lower-capacity models to be used effectively.Ī very popular representation is the so-called piano roll, which dates back to the player pianos of the early 20th century. The physical process through which sound is produced is abstracted away. Music generation has traditionally been studied in the symbolic domain: the output of the generative process could be a musical score, a sequence of MIDI events, a simple melody, a sequence of chords, a textual representation 1 or some other higher-level representation. Please don’t hesitate to suggest relevant work in the comments section! Motivation Why audio? Note that this blog post is not intended to provide an exhaustive overview of all the published research in this domain – I have tried to make a selection and I’ve inevitably left out some great work.

If you want to skip ahead, just click the section title below to go there. Finally, I’ll raise some observations and discussion points. In the next two sections I’ll attempt to cover the state of the art in both likelihood-based and adversarial models of raw music audio. Then I’ll give an overview of generative models, the various flavours that exist, and some important ways in which they differ from each other. I’ll try to motivate why modelling music in the waveform domain is an interesting problem. This blog post is divided into a few different sections. Please let me know so I can fix it! Presenting our tutorial session at ISMIR 2019 in Delft, The Netherlands.

#Auto music spectrograph song program update#
I have taken the time to update the blog software, so if anything looks odd, that may be why. This is also an excellent opportunity to revive my blog, which has lain dormant for the past four years. I’ve also added a few things to this post that I’ve thought of since giving the tutorial, and some new work that has come out since.
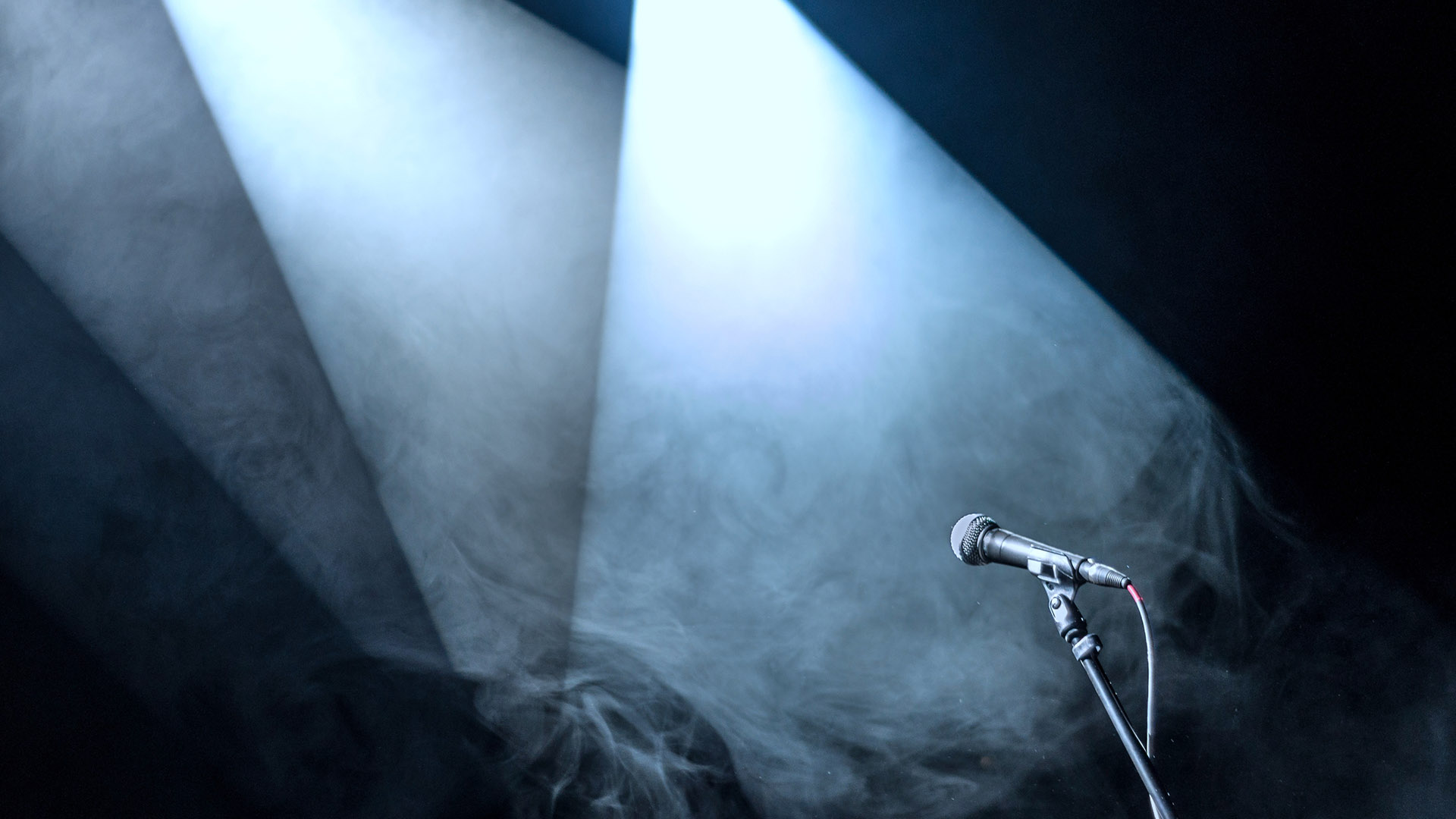
In the meantime, the slide deck we used includes all three parts and is now available on Zenodo (PDF) and on Google slides. I will share them here when they are published. Note that I will only be covering music generation in this post, but Jordi and Jongpil are working on blog posts about their respective parts. Our tutorial on the first day of the conference gave rise to plenty of interesting questions and discussions throughout, which inspired me to write some of these things down and hopefully provide a basis to continue these discussions online. With about 450 attendees (the largest edition yet), it made for a very different experience than what I’m used to with machine learning conferences like ICML, NeurIPS and ICLR, whose audiences tend to number in the thousands these days. ISMIR used to be my home conference when I was a PhD student working on music information retrieval, so it was great to be back for the first time in five years.
